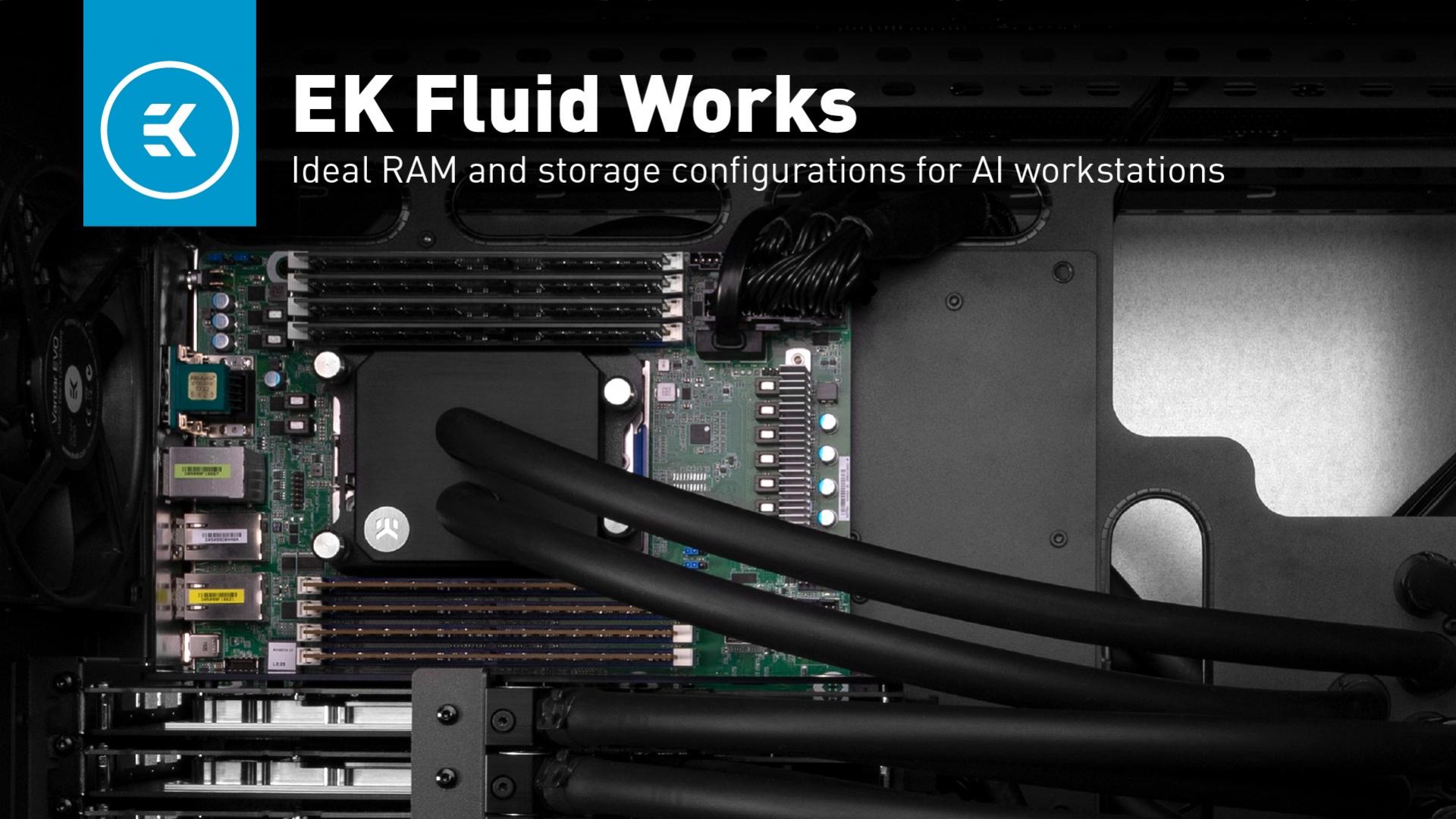
Ideal RAM and storage configurations for AI workstations
2/18/2022 10:09 AM
The ideal RAM and storage configurations for a workstation can bring countless benefits. However, if you have the wrong configurations, doing the lengthy calculations and interference modeling is taxing on all its systems. RAM and storage memory in particular can be problematic if you don’t have enough space to fit all of your working and cold data. If you don’t have the proper motherboard as well, your choices are severely limited.
Instead, learn the finer details of obtaining the correct RAM amount and speed, as well as some of the storage options available. Your work and productivity will thank you.
The right RAM for an AI workstation
The type, generation, and speed of RAM will be dictated by your initial selection of CPU and motherboard. You need to give some thought to the following:
- DDR4 or DDR5
- Frequency and latency
- Total and per DIMM capacity
The current widespread type of RAM is DDR4. It is widely available in good quantities, and there are plenty of speeds, latencies, and manufacturers to select from. Some notable options are Corsair, G.Skill, Patriot, Crucial, Team Group, and Kingston. DDR5 is the “new kid on the block” and still has some growing pains to figure out before it can officially replace DDR4. Plus, as with any new generation of DDR RAM, it is too expensive at the moment.
Source: Micron
The frequency and latency of your selected RAM is important for AI, but up to an extent. It can suffer from a severe case of diminishing returns past 3600MHz at CL14 or CL16. Higher speeds and tighter latency can create additional strain on your Integrated Memory Controller (IMC), affecting your systems stability over the long term.
So, you should look for a happy middle ground. Its worth paying attention to your CPU platform’s supported speed. The default is JEDEC’s 2133MHz that every motherboard and CPU on DDR4 support, but you will only get the full advantage of your RAM by turning on the XMP. Although it is technically considered overclocking, most modern platforms support at least 3000 -32000MHz frequency.
Source: Crucial
One more thing that could directly affect your workflow and the system stability is the RAM’s overall capacity. In general, you should consider obtaining 30-50% more RAM than GPU VRAM, so that you don’t run into any bottlenecks. When you expertly select your GPU, it’s important your RAM complements it. However, no matter how much GPU horsepower you end up with, it is ill-advised to go below 32GB.
Source: AsRock
Want to reach higher capacity numbers above 128GB without breaking the bank? U Your best bet is to look at moving to one of the higher-end platforms like Intel Xeon or AMD Threadripper. They support a higher number of memory channels in addition to their motherboards usually featuring more than the default 4 slots. This will make your life a lot easier if you are in need of more working memory capacity for your AI projects. Some of our EK Fluid Works Compute series workstations support up to 768GB of DDR4 RAM.
All the storage for your deep learning needs
Storage options can vary to a large extent depending on your AI workflows needs. There are several factors to consider here:
- Available budget
- Local vs downloaded data storage (for example, from the cloud or server)
- Available space inside the case and connections on the motherboard
- The general size of your data sets
Source: Samsung
You’ll definitely want an SSD to be your main OS drive and, if funds allow, as much SSD storage as possible. They are several times faster than hard drives and consume less power and space. However, they cost more per GB compared to HDDs. A well-balanced configuration would be at least 2 SSDs, 1-2TB in size. One should be used as the main OS drive and the other to serve as a cache or scratch disk. Then the rest of the storage array can be comprised of hard drives 8 to 10 TB each.
Serve The Home
If you have access to a high-speed internet connection, cloud storage is a solid choice. However, a NAS solution is a more viable and easily expandable option. Having enough SATA ports and M.2 slots is also worth considering when picking the right motherboard.
M.2 drives will be bolted directly to the motherboard and you will want at least two M.2 slots on the motherboard. SATA ports are more compact and a motherboard will usually have 6 or more. The number of ports, along with the workstation’s case, will be the main limiting factor on how many HDDs\SSDs you can fit inside it. How much space you need depends on the size of your data sets.
Do you have a larger data set? You can further expand the number of available ports, be it M.2 or SATA, by using PCIe adapter cards. But this will eat into the number of PCIe slots available for the GPUs. So, it is a balancing act. It can understandably be stressful finding the right configuration, so there is another option. If you prefer to have a workstation that is already professionally configured, it’s worth checking out the EK Fluid Works Compute Series X5000 workstations. It offers enough flexibility and a good chunk of computational muscle to get you started.
When it comes to reputable HDD manufacturers, there aren’t many left. Your best choice is between Western Digital, Seagate, and Hitachi. For SSDs, you can add Intel, Samsung, Kingston, ADATA, and Crucial to the list.
Now you are armed with all the knowledge and tips needed to build a well-balanced storage solution for your machine learning workstation. Make sure to follow-up on our future articles as we will be rounding off the journey with the key subsystems like the PSU, cases, and more!